Common Geocoding Mistakes and How to Avoid Them
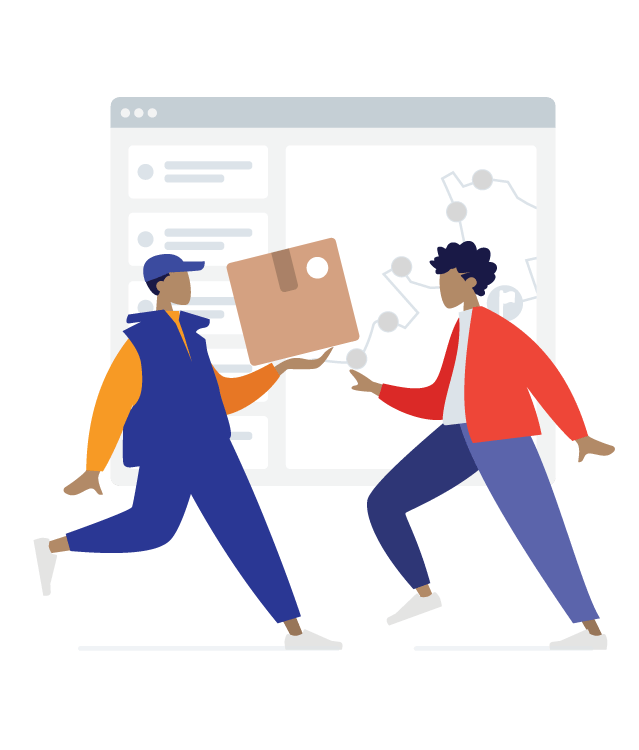
Reduce costs and maintain quality of service with AI-based geo-coding
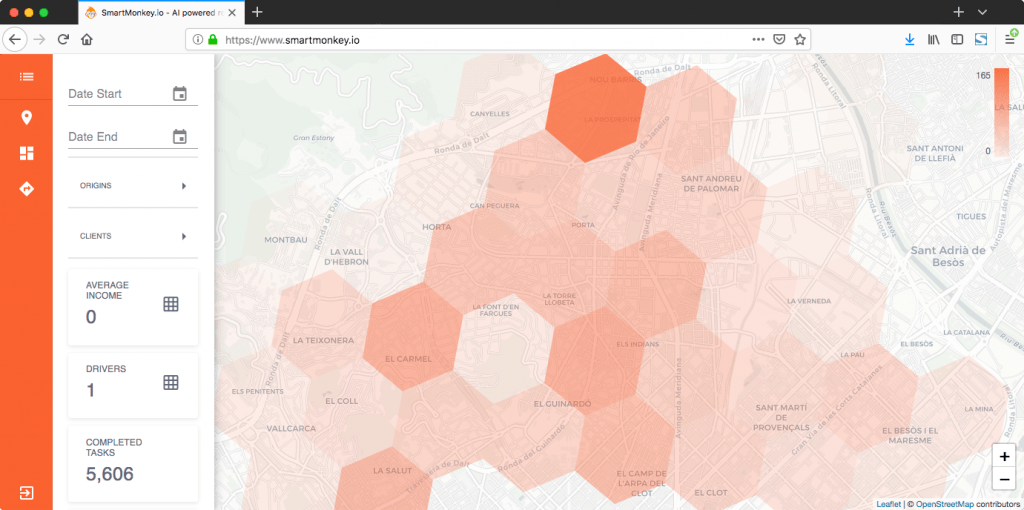
Hello, I'm Xavi Ruiz, CEO of Routal . Last week I was talking to Muthu about Geoawesomeness About what we do in Routal. During this conversation, I commented that non-geographic specialists have problems due to their lack of knowledge about geographic coding systems. They think it's no longer a problem, so when you talk to them about the quality of their master data they say: “Let's go! We have Google Maps, right?” It's a normal reaction!
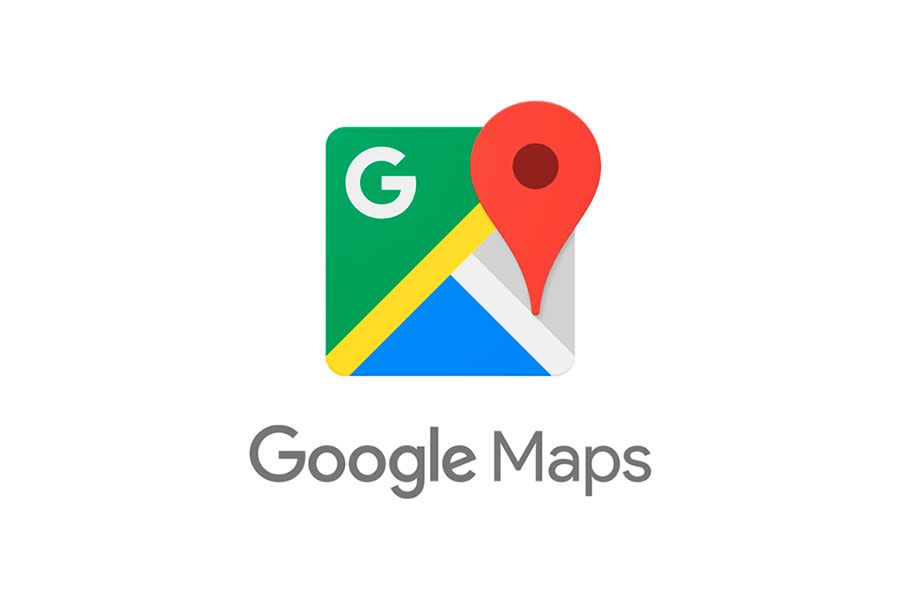
The magic wand of geocoding
Google has a good reputation as a technology giant and works well in urban settings. But if we ask them “Have you ever gotten lost using Google Maps?” triggers bad experiences in their minds. I'm a big user of Google Maps and I can identify with that.
The main question that comes up is why do Google Maps users trust their geocoding so much?
Geocode , for non-experts, means providing the geographical coordinates corresponding to a location.
They trust Google Maps for their expertise. They don't understand how it works and for them it's magic: they enter an address and Google magically puts a pin on a map. The magic feels perfect!
But the bad news is that Google Maps is far from perfect.
A study by the University of Pittsburgh (2009) on market quality concludes that geocoders do not offer sufficient quality. In short, 60% of the data is accurate enough (< 200 m), 20% has enough error, which is useful for a route optimizer, and the rest is not even a result (manual geocoding is still necessary) .* (See reference at the end of the post)
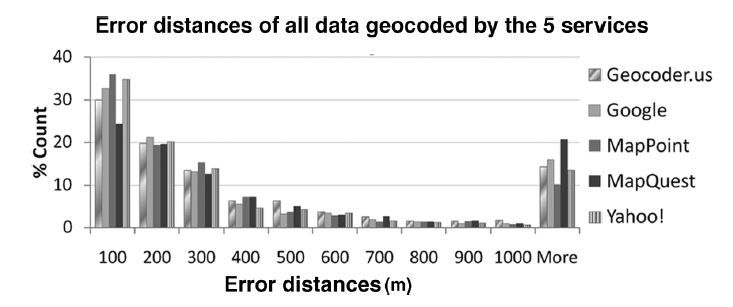
Achieving 60-70% correction is far from magical or perfect.
Logistic operations cannot be based on results with an error close to 30% in geolocated locations.
When geocoding meets reality
Why isn't Google working? magically ? There are several reasons:
- The main reason is the lack of information in the field compared to urban areas.
- Another reason is that names change. The administration changes the name of streets, squares and avenues over time. Information must be up to date. And the system should keep in mind that users can still search for old names when they think of a place. Sometimes places have cozy names that are familiar to the townspeople. But these places don't exist for Google Maps.
- Multilingual regions they have different translations and multiple combinations for the same place.
Living in the Non-Existent Star
I have a personal story to tell as an example of this problem with Google Maps.
My wife lived in a town near Girona called Olot. This region is famous for its political majority towards Catalan nationalism. My wife lived in a square officially called “Plaça Espanya”. To avoid this name, the inhabitants of Olot refer to it with the cozy name of “Plaça Estrella”. There's no need to ask why.
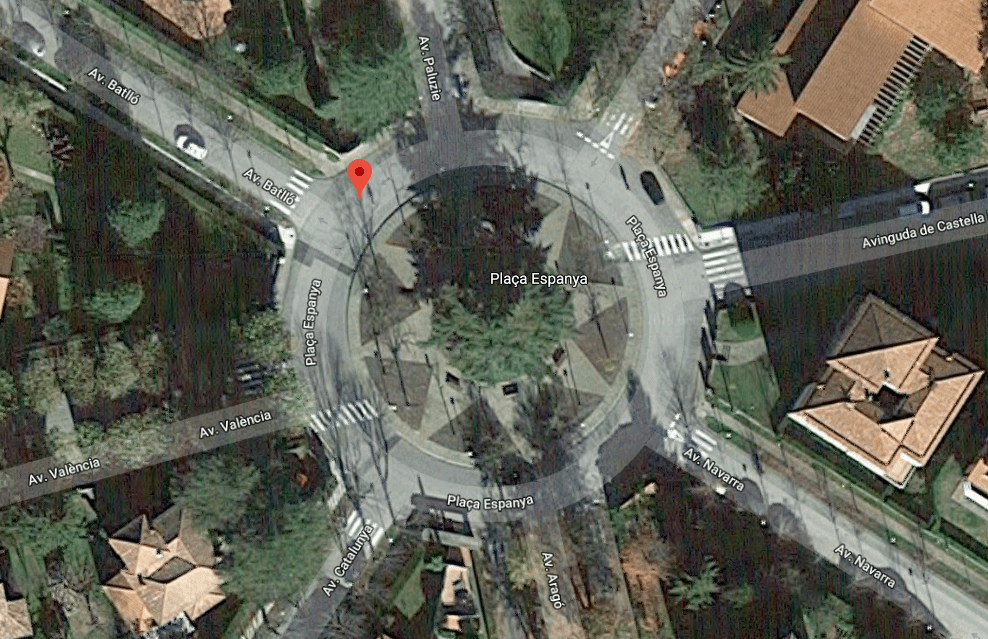
Now you can imagine the enormous problems that DHL or UPS workers had to deliver to this square!
Trust in human knowledge
How are people currently solving this problem? Obviously making mistakes and learning from them. It's that simple because none of us were born with full knowledge. Knowledge about places is acquired and shared between people. Human knowledge fills information gaps.
Since people make mistakes, 100% quality service cannot be achieved with this method.
Loss of information
Not only do workers make mistakes, besides, they aren't always available. The efficiency of operations is highly dependent on experienced people. This shared knowledge is one of the most valuable assets of a logistics company. They realize the value of their workers when they have to replace them. It is impossible to replace knowledge and at the same time maintain a consistent level of service quality.
This is a huge risk for companies that are not aware of this problem.
Artificial intelligence to the rescue
Artificial Intelligence is able to learn from operations as humans do. It learns automatically, without errors, and AI is always available. Routal helps companies in their logistics operations and reduces their costs by up to 30%.
Case Study: Suez
Suez is the world's largest utility company. As a water supplier, they have hundreds of thousands of places they visit periodically: water meters, valves and others.

Case Study: Suez
In some remote areas, there is only one person responsible for all of the company's operations. A single person has the knowledge of geolocation. Finding these water meters and valves is a real challenge. Google Maps won't be of any help.
Suez faced the problem when it was necessary to replace a worker. Routal demonstrated that AI helps maintain service quality and reduce risk, time and money.
How did we do that? Routal processed the GPS route of Suez logistics and combined it with commercial data (water meter readings) to automatically geolocate the places to visit. The result was:
- More than 99% of the locations were geolocated with a maximum distance accuracy of 25m.
- 60% with a maximum distance accuracy of 2m
The automatic capture of knowledge makes information more reliable and provides clear information about the operation.
Routal helps companies overcome challenges when it comes to geolocating and optimizing routes.
Visit our website to learn more about our solutions.
Stay tuned for our next article on human behaviors!
* * * Reference * * *
Roongpiboonsopit, D. and Karimi, HA , 2009. Evaluation and comparative analysis of online geocoding services. International Journal of Geographic Information Sciences , 24 (7), pp. 1081-1100. Source: https://www.tandfonline.com/doi/abs/10.1080/13658810903289478